Peregrine Falcon (Falco peregrinus)
Nesting Habitat Suitability Model
for the Shasta-Trinity National Forest
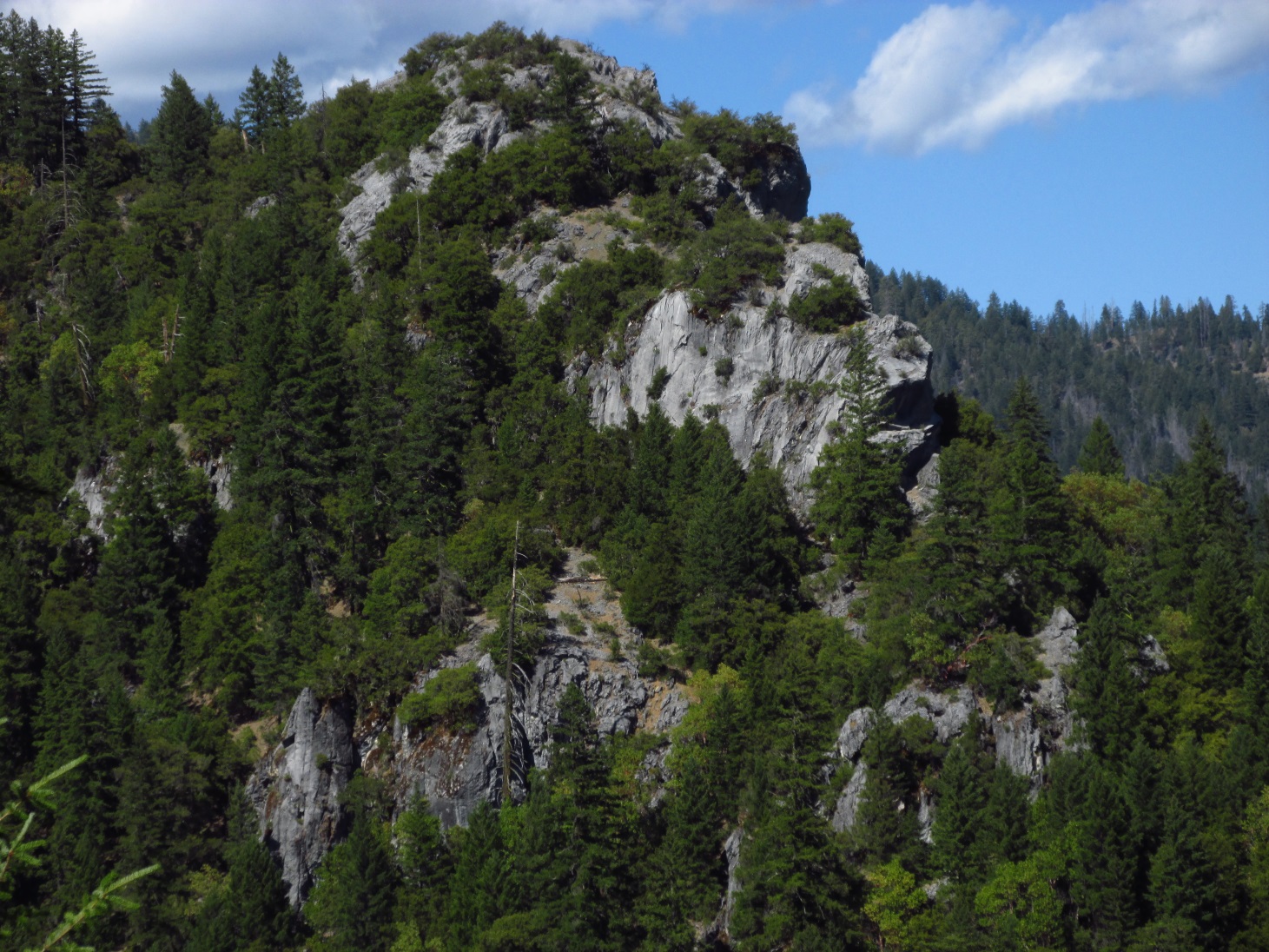
 
2017 ©William Thein
|
Author
Dale Jackan
American River College, Geography 350: Data Acquisition in
GIS; Fall 2017
|
Abstract
There have been little research on Northern California
populations of Peregrine Falcons (Falco peregrinus) in
National Forest. This project was an attempt to help
focus survey efforts in the Shasta-Trinity National
Forest (STNF). I was provided with Peregrine Falcon nest
locality data by a raptor biologist at the
Shasta-Trinity National Forest. A Maximum Entropy Model
and the Maxent program to create the habitat suitability
model (HSM) due to the small presence sample. Ten
environmental variable as potential predictors of
Peregrine Falcon nesting habitat. The variables were
slope, roughness index, distance from roads, wind
exposure index, distance from railroads, heat load
index, distance from trails, distance from lakes,
elevation, and aspect. The resulting model showed that
slope, roads, and terrain heterogeneity (roughness) were
the strongest indicators of Peregrine falcon nesting
habitat. Unfortunately this model has low predictive
value due to the elimination of many locality data
points as a result of quality control standards.
|
Introduction
There is a fair amount of knowledge
with regard to Northern California populations of
Peregrine Falcons due to it's previous listing as
Endangered under the California Endangered Species Act
CESA) and implementation of a recovery program
( Comrack
et al. 2008). There are known nesting localities
recorded by the US Forest Service (USFS) within the
Shasta-trinity National Forest (STNF), but to my
knowledge Peregrine Falcon nesting habitat suitability
modeling has not been done to determine suitable
nesting sites throughout the STNF and some of the
surrounding area. An off hand comment to the author
about desiring a model for possible Peregrine Falcon
nesting sites by a USFS raptor biologist at the STNF
prompted the attempt at creating a model. A Maximum
Entropy modeling approach was used to predict
potential Peregrine Falcon nesting habitat using the Maxent
program with 10 environmental variables.
|
Background
Preferences by Peregrine Falcons may vary with region, but
a few known factors appear to be fairly consistent across
their range. They prefer to have open habitat near nesting
sites, they nest on cliffs, and are near water (Barnes
2011, Comrack
et al. 2008). Some of these associations may be
related to their hunting behavior and water may attract
prey. I will note that peregrines do not only nest on
cliff faces and worldwide in some places tree nesting was
more widespread prior to DDT contamination (Comrack
et al. 2008) (Brambilla
et al. 2006). Given my small presence sample size a
Maximum Entropy Model was used to create a suitable
nesting habitat model for the Shasta-Trinity National
Forest and some of the surrounding area.
|
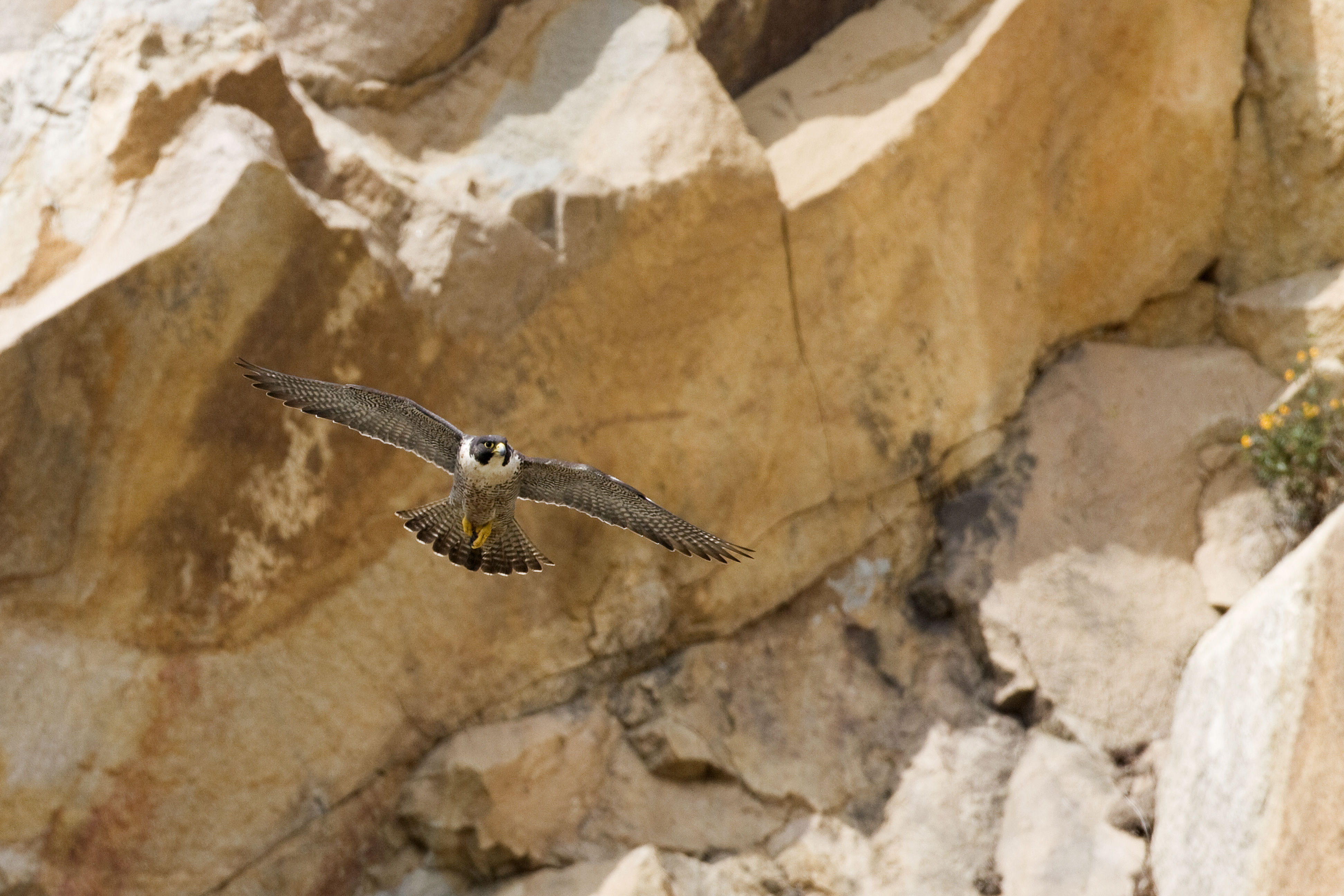
Kevin Cole from Pacific Coast, USA
(https://commons.wikimedia.org/wiki/File:Peregrine_Falcon_in_flight.jpg),
„Peregrine Falcon in flight“,
https://creativecommons.org/licenses/by/2.0/legalcode
|
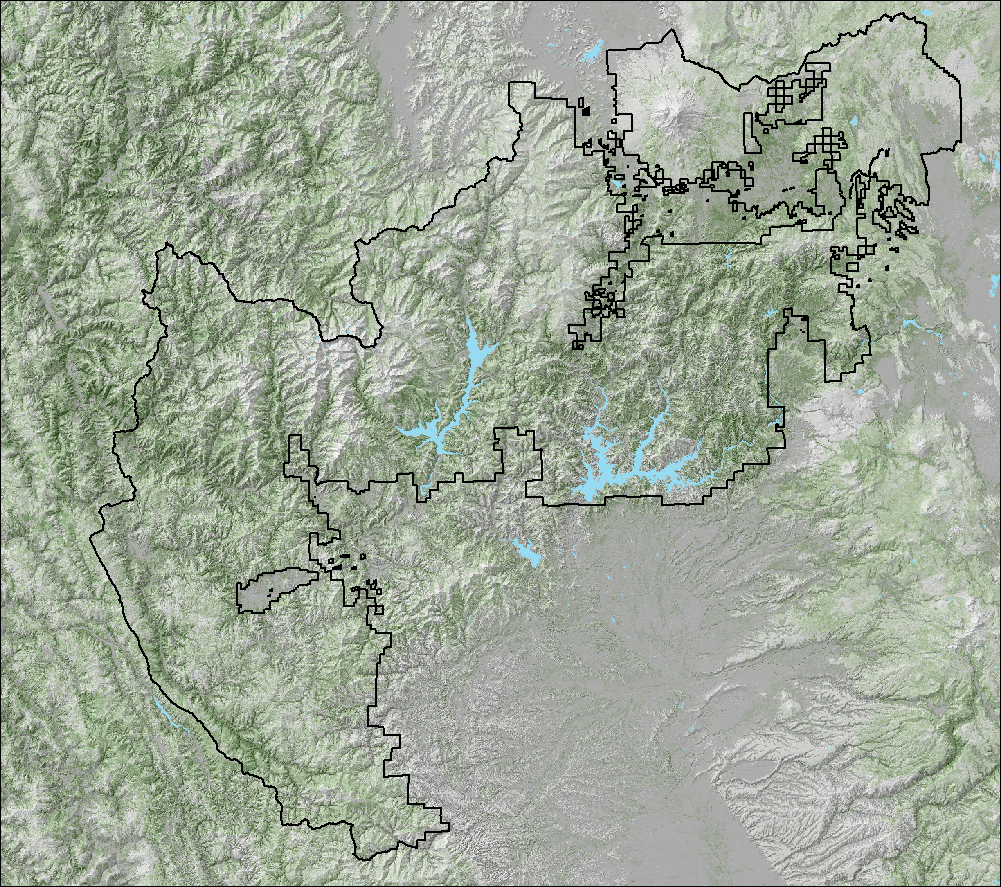
|
Methods
With the Peregrine Falcon nesting site
localities provided by William Thein of the United
States Forest Service (USFS) I began conducting
quality control checks on locality points using google
earth and the photos provided of the nesting sites
where provided. After throwing out points due to
quality control issues there were 7 nesting site
localities left. Due to the low presence sample size a
Maximum Entropy Model was used to predict possible
Peregrine Falcon nesting site habitat (Hernandez et.
al 2006).
Ten environmental variables were
selected for this model using various Geographic
Information System (GIS) programs such as ArcGIS
10.5.1, and free Open
Source programs such as SAGA
6.0.0 and
Maxent 3.4.1 ( Phillips
et. al. 2017 , Conrad et.al. 2015). Aspect was
included because it may influence heat accumulation at
the nest site and possibly wind protection, the raster
was developed in ArcGIS using the Aspect (Spatial
Analyst)tool. Slope was an included as a variable due
to it's association with cliff faces, this raster was
developed in ArcGIS using the Slope (Spatial Analyst)
tool. A wind exposure index (exposure) was included as
a variable because the nesting site may need to be
sheltered from the wind, the SAGA GIS tool Wind
Exposition Index was used to create this raster Conrad
et. al. 2015). A 5m NextMap DEM was provided by Paul
Veisze for use in the class Data Acquisition (Geog
350) at American River College for these class
projects. The DEM was used as an elevation
variable in the model. Heatload was including as a
variable because it has been suggested that day time
temperatures at the nesting site may influence their
location ( Barnes
2011). A heat load index was calculated with
ArcGIS using a 3rd
party ArcGIS toolbox (Evans et. al. 2014).
Distance from roads (roads) raster was created using
the ArcGIS Euclidean Distance(Spatial Analyst) tool).
Distance from trails (trails) was created using the
ArcGIS Euclidean Distance (Spatial Analyst) tool).
Distance from railroads (rxr) was created using the
ArcGIS Euclidean Distance tool. This layer was under
went manual quality control comparison using Google
Earth imagery for removal of abandoned railroads.
Because of the association with permanent bodies of
water a lakes layer (lakes) was created using the
ArcGIS Euclidean Distance (Spatial Analyst) tool. Two
different lakes layers were compared and the missing
lakes were added to a layer with the ArcGIS union
tool. A roughness Index (rough) was calculated to help
identify cliff face areas that are suitable nesting
habitat (Evans et. al. 2014). Slope is not always
indicative of a cliff and a roughness index would
possibly aid in the assessment of where cliff faces
are. A 3rd
party ArcGIS toolbox was used to create this
layer.
A nDVI/ nDSM layer was going to be used as a
measure of openess/canopy height, but the values
appeared wrong. I double checked and used the process
nDSM = DSM - DTM using a raster calculator. The
resulting nDSM again had negative values which in an
ideal situation should never happen. Some error is
expected possibly up to a 9m error due to differences
is where the laser hits the crown and slope ( Duan,
Z. et. al. 2015). The negatives on a
histogram were distributed well beyond
the 9m point with a minimum value of -130m. The
negatives made up 12% of the entire nDSM. When plotted
the negatives strangely plotted on some of the high
elevation areas such as Mount Shasta essentially
visually making it a caldera. Later
I did take the negatives and make them positive with
the raster calculator using CON(rasterX < 0,
rasterX * -1, rasterX). The resulting pattern did seem
to match expectation, but still considered too dubious
for use in the model. However the resulting layer was
suitable for visualizations of the area. This variable
was thrown out and will eventually be included once a
replacement is found.
In order to create a model I chose the maximum entropy
model approach due to the very small sample size and
presence only data points (Hernandez et. al 2006). To
create this model I used the Maxent
program (Phillips S.J. 2017). Maxent has several
constraints on data input that required significant
storage and processing time. Some the requirements for
data input are all layers must have the same extent,
have the same projection, and have the same cell size.
All layers must be in one of the following file
formats .asc, .gri, .mxe. Locality points need to be
in a carefully formatted according to a specific
format and saved as a comma delimited.csv file. The
created raster layers were clipped using USFS boundary
polygon for the Shasta-Trinity National Forest with an
added 10km buffer. The ArcGIS clip (data management)
tool for rasters was used with the "maintain extent"
option. In ArcGIS the resample (data management) was
set to a larger 5x5 cell size to ensure a match among
all layers. Rasters were then converted with ArcGIS
"Raster to Ascii" tool to the .asc file type. When
dealing with large files in ArcGIS and doing
conversions save the output in the same folder as your
source/input. I found if I did not do this ArcGIS
wouldn't process the layer and give some sort of
coding error. In some cases this did not work and
recalculating the statics for the layer would fix the
issue. After hours of processing I was met with a
"java heap error" because Maxent ran out of memory
because MS Windows 10 can only allocate 1.3G of RAM of
continuous RAM to the program under java. The work
around was to run the program under Linux which does
not have this limitation and in the script file (.sh)
set the memory allocation to near your max system
memory. If you run into this error look to see if
there is a "maxentcache" folder with your layer files
converted to .mxe if so just use those as your layer
files they are smaller and easier on memory usage.
|
Results
|
|
|
Figures and Maps
|
Variable |
Percent
contribution |
Permutation
importance |
slope |
34.3 |
42.1 |
rough |
31.3 |
0 |
roads |
21.2 |
55.2 |
exposure |
6.4 |
0.1 |
rxr |
2.7 |
2.4 |
heatld |
2.1 |
0.1 |
trails |
2 |
0 |
lakes |
0 |
0 |
dtm |
0 |
0 |
aspect |
0 |
0 |
|
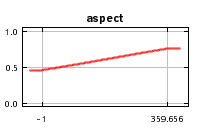 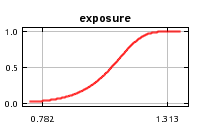 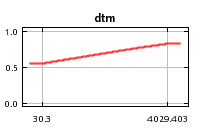 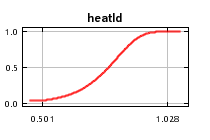 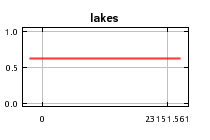
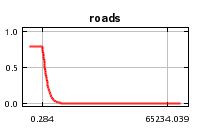 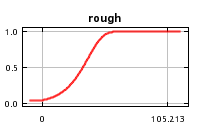 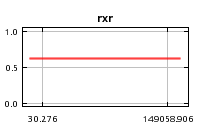 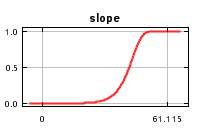 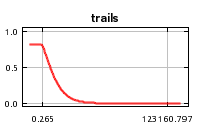
|
Figure 1.
Represents the contribution each variable makes to the
model. The permutation importance represents the gain as
the model is fitted.
|
Figure 2. These
are the models response curves to each variable. More or
less it gives you an idea if the response was positive or
negative and how quickly the response was.
|
|
Variable |
Fitted-lambdas
|
Minimum
|
Maximum
|
Aspect |
0.0
|
-1.0
|
359.6556091308594
|
dtm
|
0.0
|
30.299999237060547
|
4029.403076171875
|
Exposure |
5.649085624345769
|
0.7818626165390015
|
1.3125859498977661
|
Heatld
|
0.5116726733240735
|
0.5014203786849976
|
1.0279760360717773
|
lakes
|
0.0
|
0.0
|
23151.560546875
|
roads
|
-51.68423083717352 |
0.2838197946548462
|
65234.0390625
|
rough
|
0.0
|
0.0
|
105.21299743652344
|
rxr
|
3.4626300584649505
|
30.27614974975586
|
149058.90625
|
slope
|
12.409922976119857
|
0.0
|
61.11526107788086
|
trails
|
-1.3919254364894016
|
0.26538679003715515
|
123160.796875
|
|
Figure 3. This is
a jackknife chart essentially where the blue informs you
of how important the variable to the model by itself. The
teal color informs you of the model response if that
variable is removed. If there is not much of a reduction
in gain with it's removal most likely there is some
correlation among variables.
|
Figure 4. The most
important part of this chart are the fitted_lambdas.
Essentially they inform you of how the model responds to
the variables. If there are negative values there is a
negative response and if positive values the response are
positive. These values also give some indication of the
weight given to each variable.
|
|
|
Analysis
Of the ten variables used in this model seven appear to
have some contribution to the model (fig.1). Slope has the
highest contribution followed by the roughness index and
roads. The three variables that had no contributive value
to the model were lakes, elevation, and aspect.
The slope had the highest contribution and strongest
predictive value among all of the variables with a
moderate positive sigmoidal response as slope increased,
and the largest decrease in gain after removal from the
model (fig. 2,3). Given the preferred nesting habitat is
on cliff faces this makes sense (Barnes 2011, Comrack and
Logsdon 2008).
The roughness index showed a strong positive sigmoidal
response as roughness increased( fig. 2, fig.4). Basically
this is a measure of topographic heterogeneity and given
that cliff faces are atypical for the area I would expect
areas with higher heterogeneity to include greater slope
variation. The removal of the variable from the model had
little affect on the gain and is therefore highly
correlated with other variables (fig. 3).
For roads there was a moderate negative sigmoidal response
curve as distance from roads increased (fig.2, fig. 4).
Roads do show a strong positive response to being in the
model and show some response to their removal (fig.3).
Though that may mean it is an important variable this is
most likely the result of sample bias and more presence
samples are needed to clarify the response. This is also
where the understanding of Peregrine falcon behavior
becomes important and an understanding of how the data was
collected. The bias is most likely due to roads giving
easier access to remote areas and therefore more sightings
near roads. The subject matter expert (SME) who provided
the presence samples did inform me that some raptors
actually do have a positive response to roads due to
greater availability of perches near an open area, and may
even scavenge road kill.
For the wind exposure variable there was a weak positive
sigmoidal response as wind exposure increased (fig.2, fig
4). This is most likely due to correlation between slope
and wind exposure, there was only a small decrease in gain
as the wind exposure variable was removed from the model
(fig 3 jack). Peregrine falcons prefer very steep sloped
nesting sites, generally on cliff faces, this habitat
choice generally indicates a greater wind exposure (Barnes
2011, Comrack and Logsdon 2008).
For the railroads variable there was a positive effect
that did not change over distance (fig.2). After examining
the jackknife test the variable on it's own has no value
and should be removed from the model (fig. 3).
The model has a very weak positive sigmoidal response to
an increasing heat load index (fig.2. fig.4). Heat load
index is a relative measure of how much heat a particular
area may retain based on hours of sunlight while also
taking into account aspect, as in southwestern aspects
will generally retain more heat than all others (Evans et.
al. 2014).
For the trails variable there was a very weak negative
sigmoidal response as distance from trails increase
(fig.2, fig. 4). This is most likely a artifact of
sampling bias given sample sites are more often near roads
and trails given ease of access. Given that the gain does
not decrease after exclusion form the model more presence
samples are needed to clarify the response (fig. 3).
|
Conclusions
Unfortunately this model has low predictive value due to
the elimination of many locality data points as a result
of quality control. However stricter quality control
standards are now being implemented for data collection in
the Shasta-Trinity National Forest and the variable layers
still hold value having been created and processed for
habitat modeling. If a protocol or structured survey
methodology is implemented in the STNF with modeling in
mind then additional locality points will improve the
accuracy of this model. This project makes no attempt to
assess the accuracy of the model. A lot of models base
there data points on presence or observation points of a
species, as does this model. The difference for this model
is the unit of study is the nest locality, not where a
peregrine falcon is observed. What this means is naturally
this model will be derived from fewer presence points
because there are always going to be fewer nest localities
as opposed to observations of individuals anywhere
spatially. In the future there are steps that can be taken
to fine tune this model such as using environmental
variable data for the species over a much larger portion
of the range and use a portion of it as background
samples. Additional suitable variables will continue to be
added as they are found, and others rejected as the
analyses dictate. Developing and improving this model will
be an ongoing iterative process as I learn new techniques,
as additional research is published, and as I develop a
greater understanding of the subjects encompassing habitat
modeling and prediction.
|
Acknowledgements
I would particularly like to thank the
following people and entities for there support and
advice on this project:
The US Forest Service and William Thein for providing me
with the nest site locality data.
Paul Veisze
Marvin Castenada
I am sure there are many other people that have helped
me along the way with this project and though I may have
forgotten to include you I thank you for your help and
support as well.
I would also like to credit free and open source
projects such as SAGA GIS,
Maxent,
and Linux ( Solus OS)
that played an important role along the way and saved my
bacon many times.
Most importantly I would like to thank my fiancée Paula
Carrico for all of her support along the way. She is
always there to lend an ear and her prespective, and do
other important things like keep me from killing my
computer.
|
References
Barnes, Joseph Graham,(2011) " An
Ecological study of peregrine falcons (Falco
peregrinus) at Lake Mead National Recreation Area,
2006-2010" .UNLV
Theses/Dissertations/Professional
Papers/Capstones.Paper 1028.
Brambilla M, Rubolini D, Guidali F, (2006) Factors
affecting breeding habitat selection in a
cliff-nesting peregrine Falco peregrinus population.
J Ornithol 147:428-435
Comrack, L.A. and R.J. Logsdon (2008) Status
review of the American peregrine falcon (Falco
peregrinus anatum) in California. California
Department of Fish and Game, Wildlife Branch, Nongame
Wildlife Program Report 2008-06. 36pp+ appendices.
Conrad, O., Bechtel, B., Bock, M., Dietrich, H.,
Fischer, E., Gerlitz, L., Wehberg, J., Wichmann, V.,
and Böhner, J. (2015): System for Automated
Geoscientific Analyses (SAGA) v. 2.1.4, Geosci. Model
Dev., 8, 1991-2007, doi:10.5194/gmd-8-1991-2015.
Evans JS, Oakleaf J, Cushman SA, Theobald D (2014) An
ArcGIS Toolbox for Surface Gradient and
Geomorphometric Modeling, version 2.0-0.
Available: http://evansmurphy.wix.com/evansspatial.
Accessed: 2015 Dec 2nd.
Hernandez, P. A., Graham, C. H., Master, L. L. and
Albert D. L. 2006. The
effect of sample size and species characteristics on
performance of different species distribution
modeling methods. Ecography 29: 773-785.
Phillips, Steven J., Miroslav Dudík, Robert E.
Schapire. [Internet] Maxent software for modeling
species niches and distributions (Version 3.4.1).
Available from url: http://biodiversityinformatics.amnh.org/open_source/maxent/.
Accessed on 2017-12-9.
Duan, Z., Zhao, D., Zeng, Y., Zhao, Y., Wu, B., &
Zhu, J. (2015).
Assessing and Correcting Topographic Effects on
Forest Canopy Height Retrieval Using Airborne LiDAR
Data. Sensors (Basel, Switzerland), 15(6),
12133–12155. http://doi.org/10.3390/s150612133
|
|
|