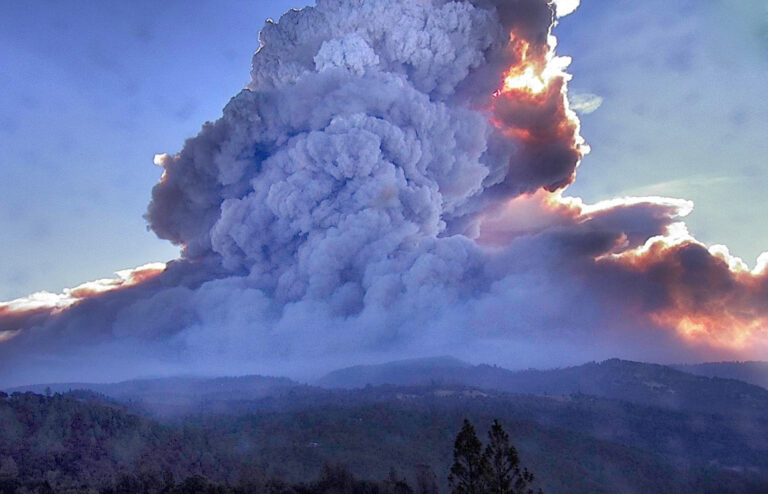
Abstract
With the growing scale of wildfire disasters in California and other western states, the need for accurate and efficient damage assessment is becoming more imporatant. Geospatial techniques and systems can be used to increase the efficiency and accuracy of the assessments. It will not completely replace "boots on the ground" assessment but can give incident command teams the information they need to better direct ground teams to more quicky create damage assessment reports allowing restoration resources to be better allocated. Newer techonolgies in deep learning and artificial intelligence are increasing being used in GIS and can be used to improve geospatial damage assessment techniques even more.
Introduction
The use of aerial imagery collected by aircraft or sUAS (drone) for wildfire damage assessment is an emerging process that will become a very important tool in the near future. With the increase in large wildfires in California and other western states this type of analysis will become more important. The results can be used to more efficiently provide aid to those affected by the disaster. Analysis can also help predict how future wildfires will progress and the damage they might cause. This will help us plan and perhaps design better defenses from these disasters.
Background
Aircraft can be used to collect aerial imagery of a disaster area to be used for damage assessment. Among other organizations, The Civil Air Patrol is using specially equiped aircraft to collect imagery for this purpose (CAP News, 2018). Once this imagery is collected, it can be used in different ways for damage assessment. Geospatial analysts can manually compare before and after images of known structure locations. Newer technologies can be used as well, such as deep learning which can be trained to identify damage (Viswambharan, 2019). Even more exciting are more recent developments at UC Berkeley and Cal Poly, San Luis Obispo are using artificial intelligence that can identify wildfire damage without "before" imagery with over 90% success (Tucker, 2021).
Methods
Among other organizations, the United States Civil Air Patrol collects aerial imagery. This imagery can be turned into large orthomosaic raster layers to be used in analysis. The State of California and other organizations collect geospatial data about roads, structure footprints, population density, and weather. These data can be put together in an analysis of the damage caused by a fire. In particular, an analysis of an orthographic mosaic of a burn scar in along with structure footprints can help us determine what structures are destroyed, damaged and saved or not affected.
Once this imagery is collected it can be used for damage assessment of structures in the burn area. Using layers indicating where buildings are known to be, the imagery can be reviewed manually by an analyst. Typically this is done by comparing the new imagery with previously collected imagary and swipe tool. This allows the analyst to compare the structure before and after the wildfire to asses the level of damage caused by the fire.
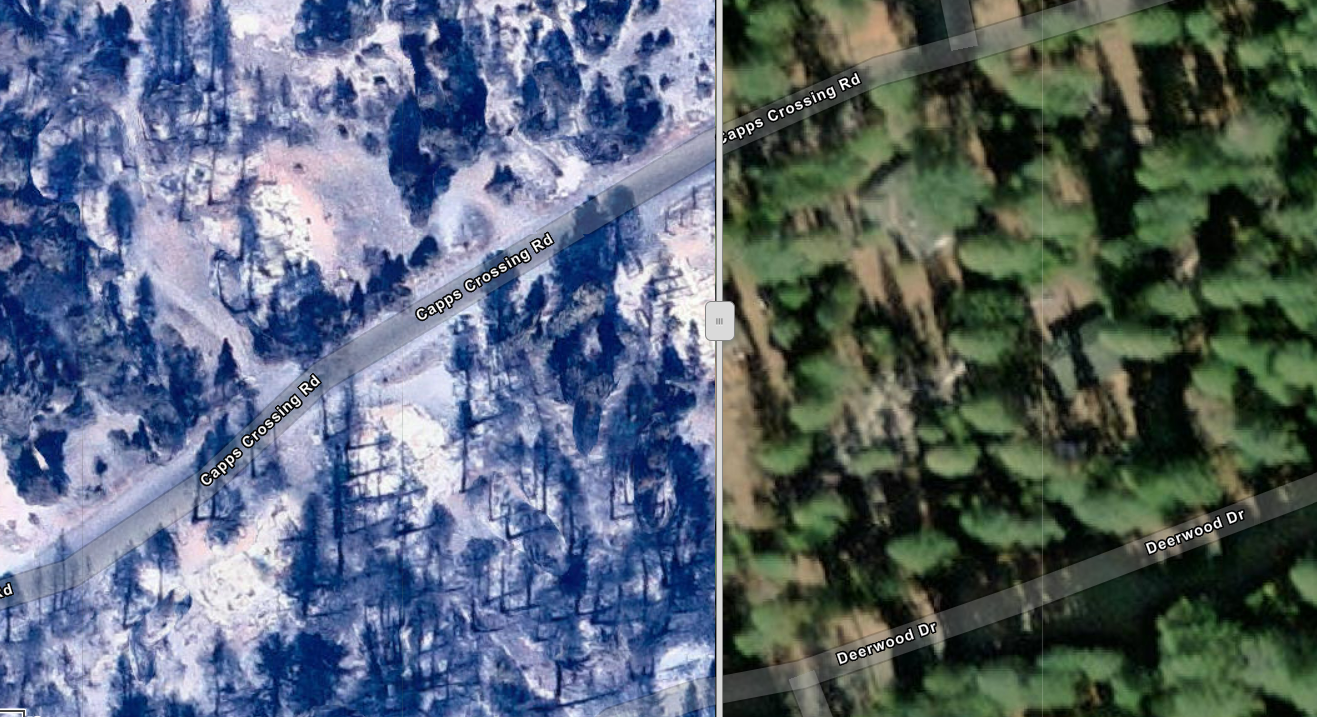
New methods of damage assessment are starting to use deep learning and artificial intelligence to expedite the process. Some processes have over a 90% success rate at identifying damaged structures without need for an analyst looking at each structure individually. In addition, these algorithms can be designed to work without "before" imagery. This is a major improvement as we do not always have imagery of an area before a disastor occurs, or if we do it may not be recently collected and may be missing newer structures.
Results
The primary data layers used for this project were:
- USA Structures (ESRI) -- Vector Polygons
- CaldorFire_2021_08_25 (Civil Air Patrol) -- Raster Orthographic Imagery
In addition the ESRI Imagery basemap was used for "before" imagary in the sample damage assessment procedures. The layers above were clipped to the area of interest, which was the extent of the aerial imagery used for this project. These layers were used in a demo applicaction created with ESRI AppBuilder and a swipe tool to simulate the damage assessment environment used by a geospatial damage assessment analyst.
Analysis
Geospatial damage assessment by a geospatial analyst using before and after imagery and know structure locations is an effective way of reporting damage assessment information to incident management teams. With a well built system, crowdsourcing can be a good way to cover large areas in a shorter time. Automated methods using deep learning and artificial intelligence will further enhace the efficiency of this process.
Conclusion
The need for "boots on the ground" damage assessment with crews going from structure to structure will not be replaced anytime soon. However these new technologies that incorporate remote sensing, GIS, artificial intelligence, and deep learning will greatly improve the efficiency of damage assessment operations for wildfires and other natural disasters. These methods can be used to identify areas of higher need and expedite response to the areas that need it most. With wildfires and other natural disasters getting larger and more destructive, the need to make efficient use of resources becomes even more important.
FEMA is already utilizing volunteer crowdsourcing for damage analysis. If you are interested in learning more or volunteering please take a look at Geospatial Damange Assessment Training, part of the FEMA Hub Community.
References
- "Specially Equipped CAP Planes Conducting Florence Damage Assessment Flights." CAP News, 20 Sep 2018, https://www.cap.news/specially-equipped-cap-planes-conducting-florence-damage-assessment-flights/. Accessed December 2022.
- Tucker, Danielle Torrent. "AI system developed by Stanford researchers identifies buildings damaged by wildfire," Stanford News, 16 Sep 2021, https://news.stanford.edu/2021/09/16/ai-system-identifies-buildings-damaged-wildfire/. Accessed November 2022.
- Viswambharan, Vinay, and Rohit Singh. "Damage assessment using deep learning in ArcGIS." ArcGIS Blog, 9 Aug 2019, https://www.esri.com/arcgis-blog/products/arcgis-pro/imagery/damage-assessment-using-deep-learning-in-arcgis/. Accessed November 2022.
- Yu, David. "Automate fire damage assessment with deep learning." ESRI Learning, https://learn.arcgis.com/en/projects/automate-fire-damage-assessment-with-deep-learning/. Accessed December 2022.